Factbox: Neuralink: what you need to know about Elon Musk's brain chip company
Neuralink, the Elon Musk company that Reuters reported on Monday is the target of a federal investigation over its animal trial program, has been trying to develop a brain chip that would enable the paralyzed to walk and the blind to see.
Here is more on what Neuralink does.
WHAT IS NEURALINK DEVELOPING?
Founded in 2016 by Musk and a group of engineers, Neuralink is building a brain chip interface that can be implanted within the skull, which it says could eventually help disabled patients to move and communicate again, and also restore vision.
Neuralink's device has a chip that processes and transmits neural signals that could be transmitted to devices like a computer or a phone.
The company hopes that a person would potentially be able to control a mouse, keyboard or other computer functions like text messaging with their thoughts.
"First @Neuralink product will enable someone with paralysis to use a smartphone with their mind faster than someone using thumbs," Musk said in April 2021.
Neuralink also believes its device will eventually be able to restore neural activity inside the body, allowing those with spinal cord injuries to move limbs. The San Francisco and Austin-based company also aspires to cure neurological conditions such as Alzheimer's and dementia.
WHAT PROGRESS HAS NEURALINK MADE?
Neuralink has produced several examples of testing aspects of its technology successfully on animals, including a video in 2021 that showed a macaque playing a simple videogame after being implanted with a brain chip. In a presentation webcast last week, the company showcased improvements in the speed and capabilities of the chip.
WHAT HURDLES FOR NEURALINK NEXT?
Neuralink has yet to secure U.S. regulatory approval to move to human trials - unlike competitor Synchron, which has less ambitious goals for its medical advances. Neuralink has missed Musk's publicly stated deadlines to start human trials and this year submitted its application to the Food and Drug Administration to begin them. Musk said last week he believes Neuralink can start human clinical trials in six months.
CONTROVERSY AROUND ANIMAL TESTING
The company is facing a federal probe for potential animal-welfare violations at a time when its staff has complained that the testing is being rushed, causing needless suffering and deaths, according to documents reviewed by Reuters and sources familiar with the investigation and company operations.
In all, the company has killed about 1,500 animals, including more than 280 sheep, pigs and monkeys, following experiments since 2018.
Source: Reuters
Factbox: Neuralink: what you need to know about Elon Musk's brain chip company
Neuralink, the Elon Musk company that Reuters reported on Monday is the target of a federal investigation over its animal trial program, has been trying to develop a brain chip that would enable the paralyzed to walk and the blind to see.
Here is more on what Neuralink does.
WHAT IS NEURALINK DEVELOPING?
Founded in 2016 by Musk and a group of engineers, Neuralink is building a brain chip interface that can be implanted within the skull, which it says could eventually help disabled patients to move and communicate again, and also restore vision.
Neuralink's device has a chip that processes and transmits neural signals that could be transmitted to devices like a computer or a phone.
The company hopes that a person would potentially be able to control a mouse, keyboard or other computer functions like text messaging with their thoughts.
"First @Neuralink product will enable someone with paralysis to use a smartphone with their mind faster than someone using thumbs," Musk said in April 2021.
Neuralink also believes its device will eventually be able to restore neural activity inside the body, allowing those with spinal cord injuries to move limbs. The San Francisco and Austin-based company also aspires to cure neurological conditions such as Alzheimer's and dementia.
WHAT PROGRESS HAS NEURALINK MADE?
Neuralink has produced several examples of testing aspects of its technology successfully on animals, including a video in 2021 that showed a macaque playing a simple videogame after being implanted with a brain chip. In a presentation webcast last week, the company showcased improvements in the speed and capabilities of the chip.
WHAT HURDLES FOR NEURALINK NEXT?
Neuralink has yet to secure U.S. regulatory approval to move to human trials - unlike competitor Synchron, which has less ambitious goals for its medical advances. Neuralink has missed Musk's publicly stated deadlines to start human trials and this year submitted its application to the Food and Drug Administration to begin them. Musk said last week he believes Neuralink can start human clinical trials in six months.
CONTROVERSY AROUND ANIMAL TESTING
The company is facing a federal probe for potential animal-welfare violations at a time when its staff has complained that the testing is being rushed, causing needless suffering and deaths, according to documents reviewed by Reuters and sources familiar with the investigation and company operations.
In all, the company has killed about 1,500 animals, including more than 280 sheep, pigs and monkeys, following experiments since 2018.
Source: Reuters
Factbox: Neuralink: what you need to know about Elon Musk's brain chip company
Neuralink, the Elon Musk company that Reuters reported on Monday is the target of a federal investigation over its animal trial program, has been trying to develop a brain chip that would enable the paralyzed to walk and the blind to see.
Here is more on what Neuralink does.
WHAT IS NEURALINK DEVELOPING?
Founded in 2016 by Musk and a group of engineers, Neuralink is building a brain chip interface that can be implanted within the skull, which it says could eventually help disabled patients to move and communicate again, and also restore vision.
Neuralink's device has a chip that processes and transmits neural signals that could be transmitted to devices like a computer or a phone.
The company hopes that a person would potentially be able to control a mouse, keyboard or other computer functions like text messaging with their thoughts.
"First @Neuralink product will enable someone with paralysis to use a smartphone with their mind faster than someone using thumbs," Musk said in April 2021.
Neuralink also believes its device will eventually be able to restore neural activity inside the body, allowing those with spinal cord injuries to move limbs. The San Francisco and Austin-based company also aspires to cure neurological conditions such as Alzheimer's and dementia.
WHAT PROGRESS HAS NEURALINK MADE?
Neuralink has produced several examples of testing aspects of its technology successfully on animals, including a video in 2021 that showed a macaque playing a simple videogame after being implanted with a brain chip. In a presentation webcast last week, the company showcased improvements in the speed and capabilities of the chip.
WHAT HURDLES FOR NEURALINK NEXT?
Neuralink has yet to secure U.S. regulatory approval to move to human trials - unlike competitor Synchron, which has less ambitious goals for its medical advances. Neuralink has missed Musk's publicly stated deadlines to start human trials and this year submitted its application to the Food and Drug Administration to begin them. Musk said last week he believes Neuralink can start human clinical trials in six months.
CONTROVERSY AROUND ANIMAL TESTING
The company is facing a federal probe for potential animal-welfare violations at a time when its staff has complained that the testing is being rushed, causing needless suffering and deaths, according to documents reviewed by Reuters and sources familiar with the investigation and company operations.
In all, the company has killed about 1,500 animals, including more than 280 sheep, pigs and monkeys, following experiments since 2018.
Source: Reuters
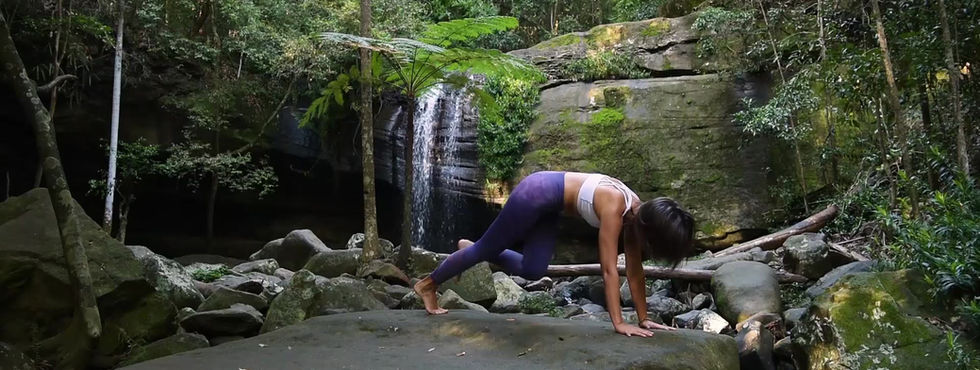
Nuero Crimes (& Nuero Assault)
Neurocriminology is an emerging sub-discipline of biocriminology and criminology that applies brain imaging techniques and principles from neuroscience to understand, predict, and prevent crime.
Source: Wikipedia
OUR DEFINITION
We believe there is much being overlooked in what is currently occurring in Nuero Crimes, such as the use of nuero devices and applications to commit crimes against people. Rather than the notion that people's thoughts and not actions constitute a crime. Such crimes include everything from bio-hacking, data mining to nuero-sexual assault through brain imaging, audio and other telepathic application of force via neuroscience. As well as the abuse and monetization of profit motivated industries such as marketing and data breaches as seen by Facebook and Cambridge Analytica. Indeed congress people and scientist alike have begun sounding the alarm for these abuses.
Nuerocriminology SOLUTIONS
WE Believe Prevention & (Religious) Intersession to be Solutions to 'crimes' that are thoughts without action. The Christian knows even their Faith is dead without works: Action. Thus we think Nuerocriminology is too preemptive.
In Hebrews 7:25 (ESV)
Consequently, he is able to save to the uttermost those who draw near to God through him, since he always lives to make intercession for them.
Life, Liberty & the Pursuit of Happiness
We have also found that these crimes (bio-technologies and technologies) as they emerge pose a serious and significant threat to life, liberty and the legitimate pursuit of happiness.
Thomas Jefferson, the Declaration of Independence
originating form Locke's inalienable rights.
May these pages empower you to life, liberty and your pursuit of happiness.
Source: Shidonna Raven Garden and Cook. All Rights Reserved.

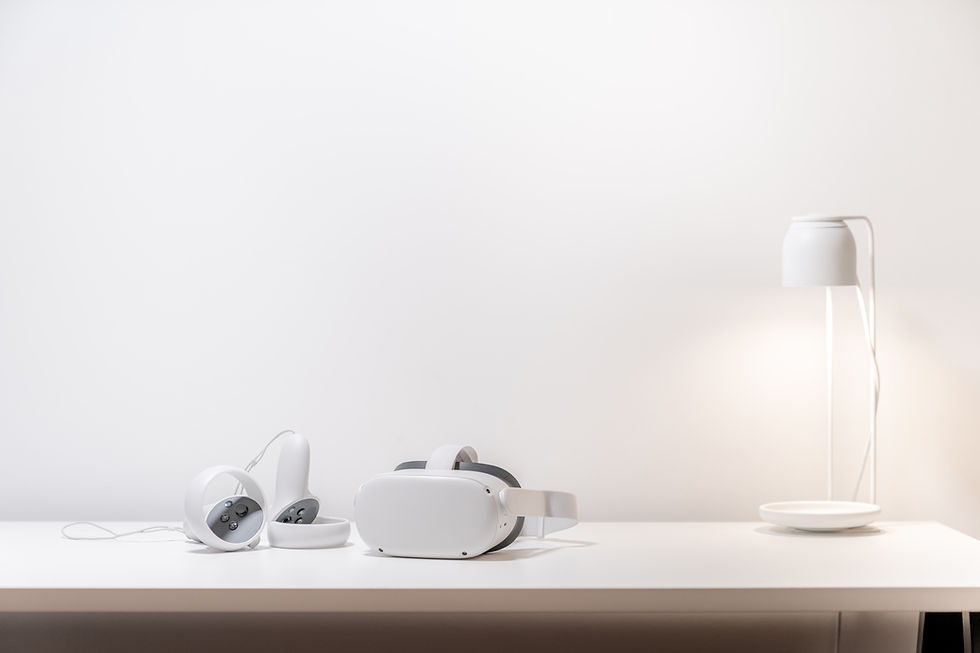
Our readers can obtain Exclusive Health Notices regarding VRUs (bio-technology) and how it pertains to Crime & Your Privacy, Protection, Property and Rights. Image by Vinicius "amnx" Amano
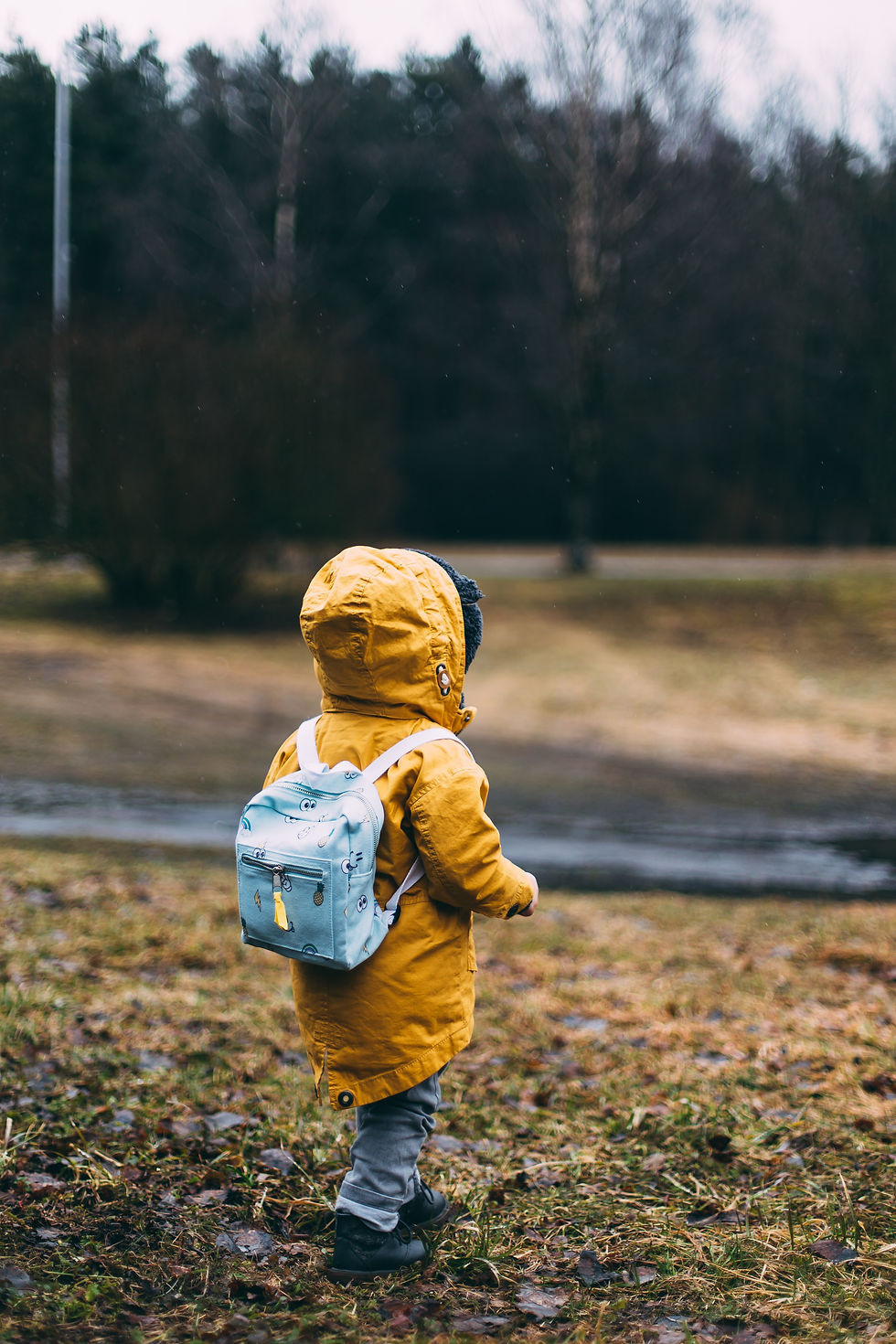
Our readers can obtain Exclusive Health Notices regarding Online Pedophiles & Other (Sexaul) Assults and how it pertains to Nuero Crimes. Image by Daiga Ellaby
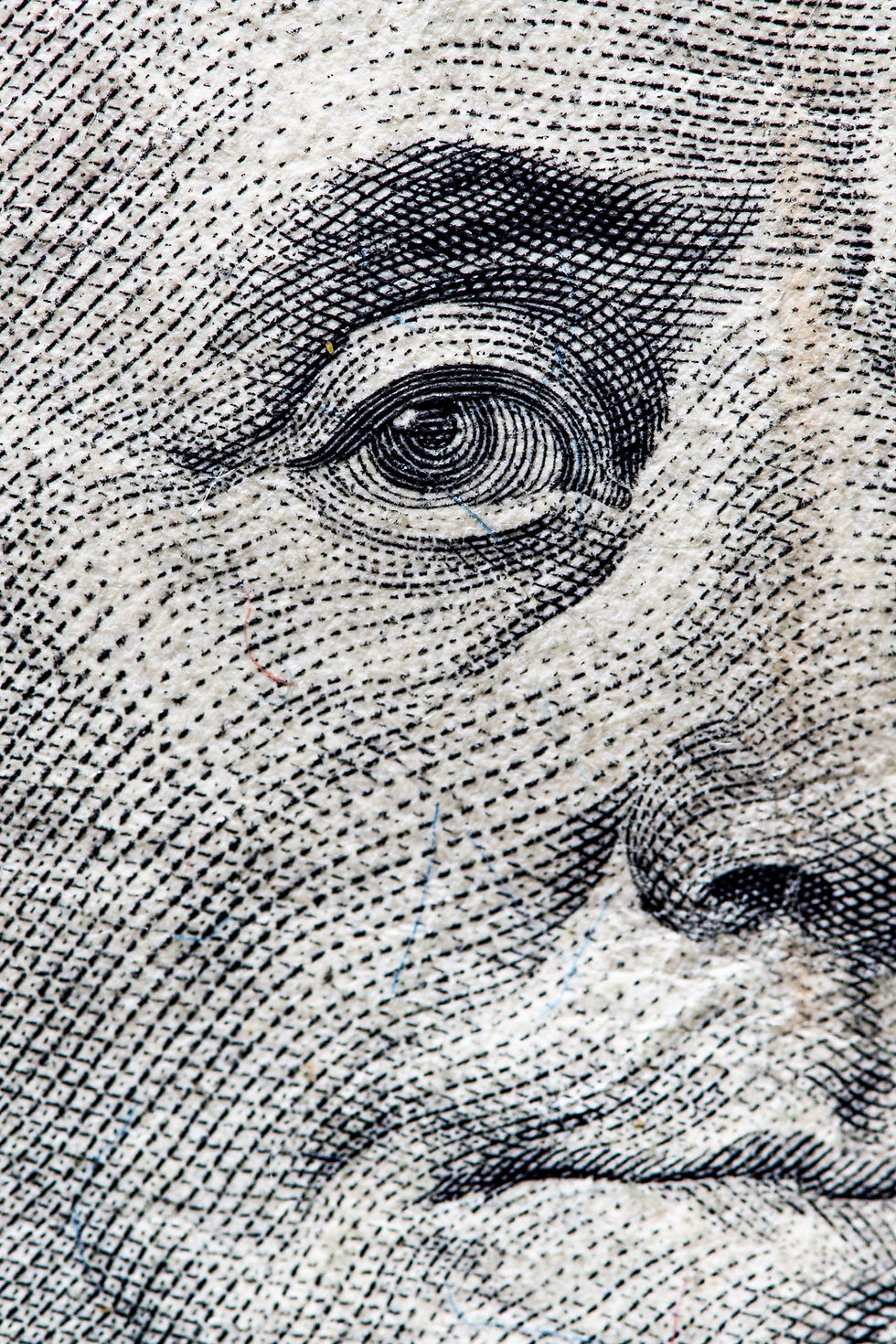
Our readers can obtain Exclusive Health Notices regarding Monitization and how it pertains to Nuero Crimes. Image by Sahand Hoseini
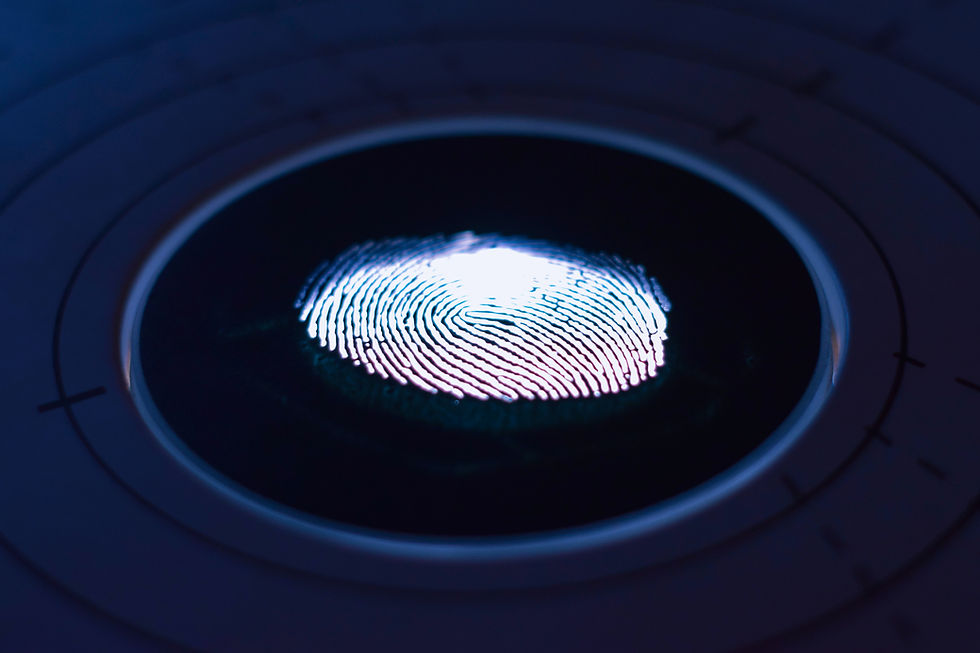
Our readers can obtain Exclusive Health Notices regarding Bio Hacking and how it pertains to Nuero Crimes. Image by George Prentzas
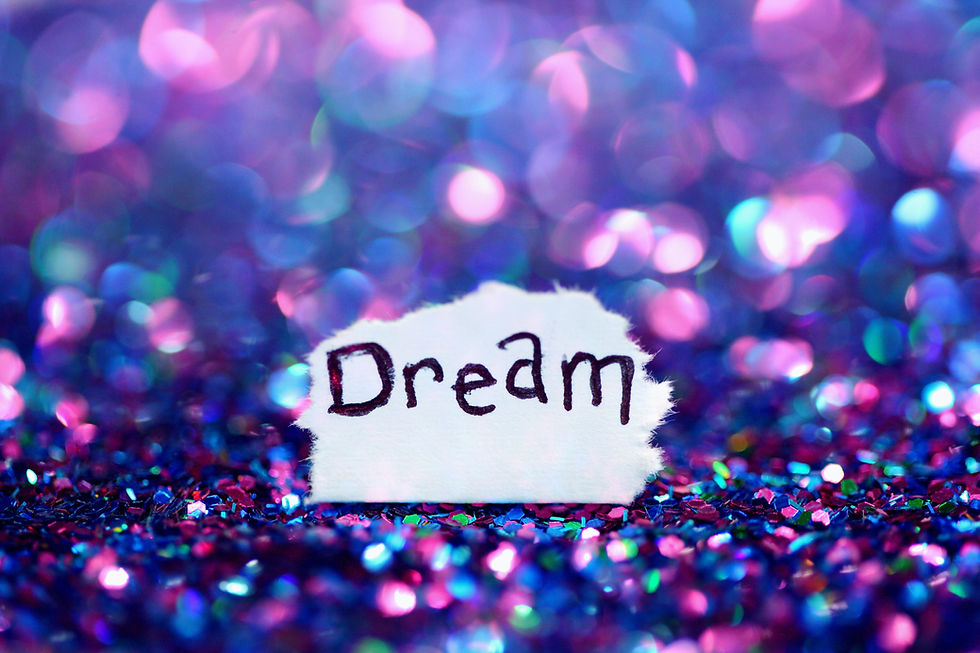
Our readers can obtain Exclusive Health Notices regarding Dream and how it pertains to Nuero Crimes. Image: Unsplash
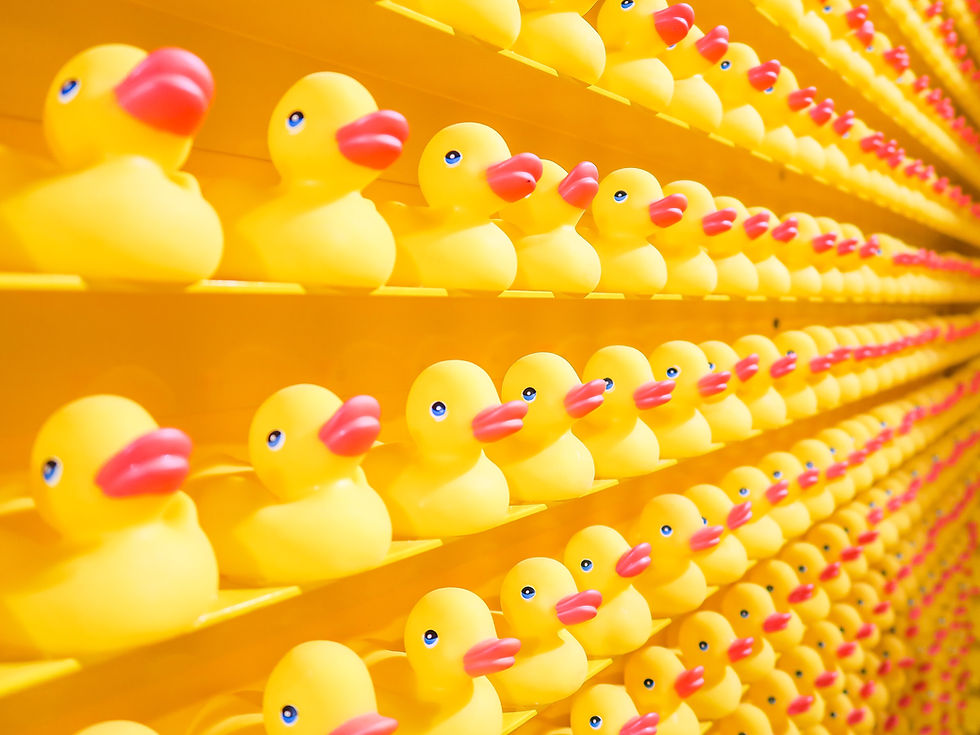
Our readers can obtain Exclusive Health Notices regarding NUEROMarketing and how it pertains to Nuero Crimes. Image by JOSHUA COLEMAN

Our readers can obtain Exclusive Health Notices regarding Audio and how it pertains to Nuero Crimes. Image by Jessica Flavia

Our readers can obtain Exclusive Health Notices regarding Healthcare and how it pertains to Nuero Crimes. Image by Abby Anaday
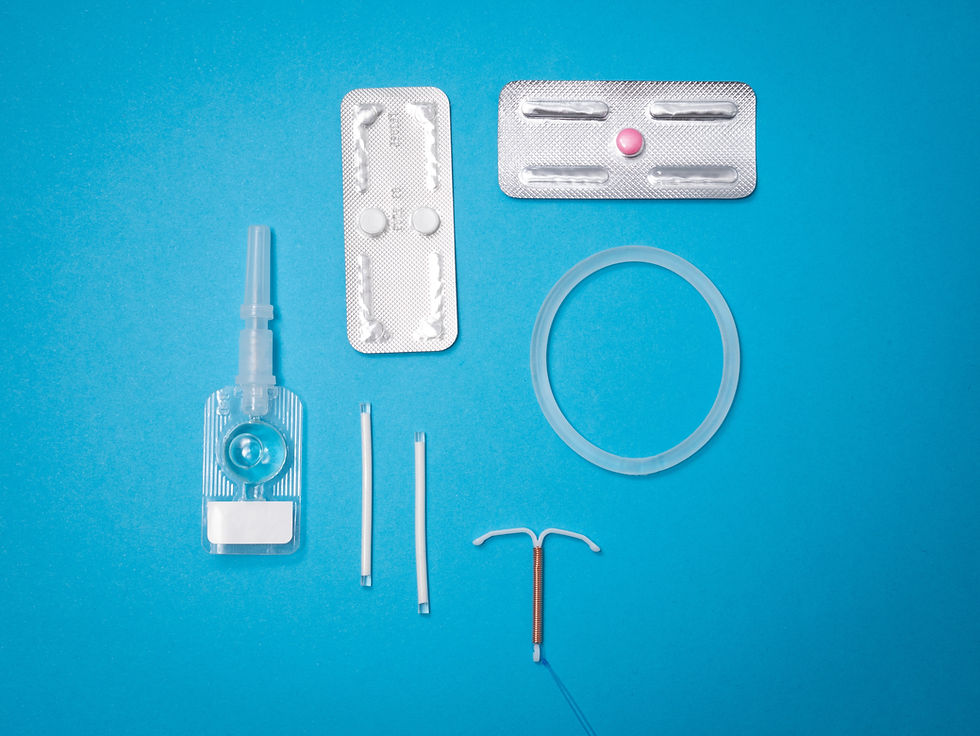
Our readers can obtain Exclusive Health Notices regarding Medical Devices and how it pertains to Nuero Crimes. Image by Reproductive Health Supplies Coalition
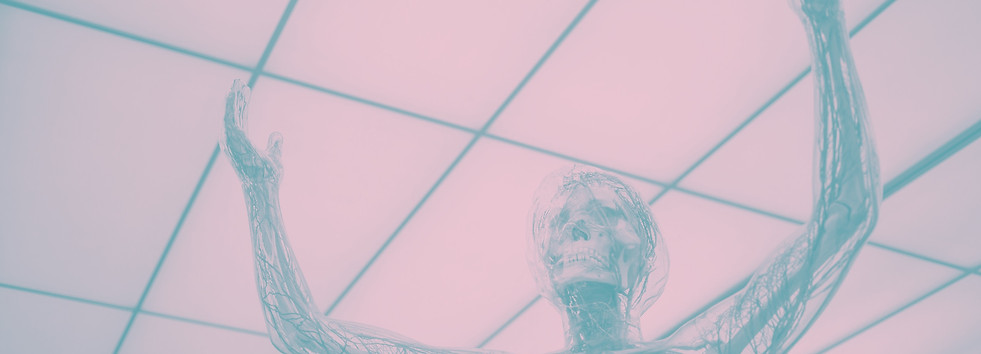
What is Nuero-Science
neu·ro·sci·ence
/ˈn(y)o͝orōˌsīəns/
noun
-
any or all of the sciences, such as neurochemistry and experimental psychology, which deal with the structure or function of the nervous system and brain.
Source: Google Oxford Language
What Are Neural Networks?
A branch of machine learning, neural networks (NN), also known as artificial neural networks(ANN), are computational models — essentially algorithms. Neural networks have a unique ability to extract meaning from imprecise or complex data to find patterns and detect trends that are too convoluted for the human brain or for other computer techniques. Neural networks have provided us with greater convenience in numerous ways, including through ridesharing apps, Gmail smart sorting, and suggestions on Amazon.
The most groundbreaking aspect of neural networks is that once trained, they learn on their own. In this way, they emulate human brains, which are made up of neurons, the fundamental building block of both human and neural network information transmission.
“Human brains and artificial neural networks do learn similarly,” explains Alex Cardinell, Founder and CEO of Cortx, an artificial intelligence company that uses neural networks in the design of its natural language processing solutions, including an automated grammar correction application, Perfect Tense. “In both cases, neurons continually adjust how they react based on stimuli. If something is done correctly, you’ll get positive feedback from neurons, which will then become even more likely to trigger in a similar, future instance. Conversely, if neurons receive negative feedback, each of them will learn to be less likely to trigger in a future instance,” he notes.
How the Biological Model of Neural Networks Functions
What are neural networks emulating in human brain structure, and how does training work?
All mammalian brains consist of interconnected neurons that transmit electrochemical signals. Neurons have several components: the body, which includes a nucleus and dendrites; axons, which connect to other cells; and axon terminals or synapses, which transmit information or stimuli from one neuron to another. Combined, this unit carries out communication and integration functions in the nervous system. The human brain has a massive number of processing units (86 billion neurons) that enable the performance of highly complex functions.
How Artificial Neural Networks Function
ANNs are statistical models designed to adapt and self-program by using learning algorithms in order to understand and sort out concepts, images, and photographs. For processors to do their work, developers arrange them in layers that operate in parallel. The input layer is analogous to the dendrites in the human brain’s neural network. The hidden layer is comparable to the cell body and sits between the input layer and output layer (which is akin to the synaptic outputs in the brain). The hidden layer is where artificial neurons take in a set of inputs based on synaptic weight, which is the amplitude or strength of a connection between nodes. These weighted inputs generate an output through a transfer function to the output layer.
How Do You Train a Neural Network?
Once you’ve structured a network for a particular application, training (i.e., learning), begins. There are two approaches to training. Supervised learning provides the network with desired outputs through manual grading of network performance or by delivering desired outputs and inputs. Unsupervised learning occurs when the network makes sense of inputs without outside assistance or instruction.
There’s still a long way to go in the area of unsupervised learning. “Getting information from unlabeled data, [a process] we call unsupervised learning, is a very hot topic right now, but clearly not something we have cracked yet. It’s something that still falls in the challenge column,” observes Université de Montréal’s Yoshua Bengio in the article “The Rise of Neural Networks and Deep Learning in Our Everyday Lives.”
Bengio is referring to the fact that the number of neural networks can’t match the number of connections in the human brain, but the former’s ability to catch up may be just over the horizon. Moore’s Law, which states that overall processing power for computers will double every two years, gives us a hint about the direction in which neural networks and AI are headed. Intel CEO Brian Krzanich affirmed at the 2017 Computer Electronics Show that “Moore’s Law is alive and well and flourishing.” Since its inception in the mid-20th century, neural networks’ ability to “think” has been changing our world at an incredible pace.
A Brief History of Neural Networks
Neural networks date back to the early 1940s when mathematicians Warren McCulloch and Walter Pitts built a simple algorithm-based system designed to emulate human brain function. Work in the field accelerated in 1957 when Cornell University’s Frank Rosenblatt conceived of the perceptron, the groundbreaking algorithm developed to perform complex recognition tasks. During the four decades that followed, the lack of computing power necessary to process large amounts of data put the brakes on advances. In the 2000s, thanks to the advent of greater computing power and more sophisticated hardware, as well as to the existence of vast data sets to draw from, computer scientists finally had what they needed, and neural networks and AI took off, with no end in sight. To understand how much the field has expanded in the new millennium, consider that ninety percent of internet data has been created since 2016. That pace will continue to accelerate, thanks to the growth of the Internet of Things (IoT).
For more background and an expansive timeline, read “The Definitive Guide to Machine Learning: Business Applications, Techniques, and Examples.”
Why Do We Use Neural Networks?
Neural networks’ human-like attributes and ability to complete tasks in infinite permutations and combinations make them uniquely suited to today’s big data-based applications. Because neural networks also have the unique capacity (known as fuzzy logic) to make sense of ambiguous, contradictory, or incomplete data, they are able to use controlled processes when no exact models are available.
According to a report published by Statista, in 2017, global data volumes reached close to 100,000 petabytes (i.e., one million gigabytes) per month; they are forecasted to reach 232,655 petabytes by 2021. With businesses, individuals, and devices generating vast amounts of information, all of that big data is valuable, and neural networks can make sense of it.
Attributes of Neural Networks
With the human-like ability to problem-solve — and apply that skill to huge datasets — neural networks possess the following powerful attributes:
-
Adaptive Learning: Like humans, neural networks model non-linear and complex relationships and build on previous knowledge. For example, software uses adaptive learning to teach math and language arts.
-
Self-Organization: The ability to cluster and classify vast amounts of data makes neural networks uniquely suited for organizing the complicated visual problems posed by medical image analysis.
-
Real-Time Operation: Neural networks can (sometimes) provide real-time answers, as is the case with self-driving cars and drone navigation.
-
Prognosis: NN’s ability to predict based on models has a wide range of applications, including for weather and traffic.
-
Fault Tolerance: When significant parts of a network are lost or missing, neural networks can fill in the blanks. This ability is especially useful in space exploration, where the failure of electronic devices is always a possibility.
Tasks Neural Networks Perform
Neural networks are highly valuable because they can carry out tasks to make sense of data while retaining all their other attributes. Here are the critical tasks that neural networks perform:
-
Classification: NNs organize patterns or datasets into predefined classes.
-
Prediction: They produce the expected output from given input.
-
Clustering: They identify a unique feature of the data and classify it without any knowledge of prior data.
-
Associating: You can train neural networks to "remember" patterns. When you show an unfamiliar version of a pattern, the network associates it with the most comparable version in its memory and reverts to the latter.
Neural networks are fundamental to deep learning, a robust set of NN techniques that lends itself to solving abstract problems, such as bioinformatics, drug design, social network filtering, and natural language translation. Deep learning is where we will solve the most complicated issues in science and engineering, including advanced robotics. As neural networks become smarter and faster, we make advances on a daily basis.
Real-World and Industry Applications of Neural Networks
As an August 2018 New York Times article notes, “The companies and government agencies that have begun enlisting the automation software run the gamut. They include General Motors, BMW, General Electric, Unilever, MasterCard, Manpower, FedEx, Cisco, Google, the Defense Department, and NASA.” We’re just seeing the beginning of neural network/AI applications changing the way our world works.
Source: Smart Sheet